728x90
그룹간 차이가 있는지 볼 수 있는 ANOVA검정과 사후검정 관련 포스팅을 해보겠습니다.
ANOVA분석
우선 분석할 내용은, 4개의 A,B,C,D라는 학급별 수학/영어/과학 점수의 차이가 있는지 분석하는 것입니다.
1
2
3
4
5
6
7
8
9
10
11
12
13
|
score_list=['math','eng','science']
from scipy import stats
fstat_list=[]
p_list=[]
for i in lg_cat_list:
fstat, p = stats.f_oneway(class_A[i], class_B[i], class_C[i], class_D[i])
fstat_list.append(fstat)
p_list.append(p)
|
cs |
기존 내용처럼 DataFrame 화하여 결과를 쉽게 볼수 있도록 하죠.
1
2
|
cat_frames={'f':fstat_list, 'p':p_list}
pd.DataFrame(cat_frames, index=score_list)
|
cs |
[output]
위의 결과에서 유추할 수 있는 결론은 수학점수에서 Class간의 차이가 있다는 것입니다.
그럼 이제 어떤 그룹간의 차이가 있는지 사후검정을 해보도록 하죠.
사후분석
사후분석 전, 분석을 위한 dataframe을 만들어 줍니다.
1
2
3
4
5
6
7
8
9
10
11
12
13
14
15
16
|
# 각 학급별 DataFrame에 group이라는 컬럼을 추가하여, 구분자를 만들어주기
class_A=class_A[['math','eng','science']]
class_A['group']=1
class_B=class_B[['math','eng','science']]
class_B['group']=2
class_C=class_C[['math','eng','science']]
class_C['group']=2
class_D=class_D[['math','eng','science']]
class_D['group']=4
# 위의 4개의 class 데이터 프레임을 concat합니다.
class_all=pd.concat([class_A, class_B, class_C, class_D], axis=0)
|
cs |
[class all이라는 테이블 형태]
사후분석을 위한 사전 준비가 완료 되었습니다.
Bonferroni method를 활용한 ttest검정을 모든 그룹간에 해
보겠습니다. (사후검정 내용)
1
2
3
4
5
6
|
from statsmodels.sandbox.stats.multicomp import MultiComparison
import scipy.stats
comp = MultiComparison(class_all['math'], class_all['group'])
# Bonferroni 방법을 통해 모든 그룹간 ttest를 진행
result = comp.allpairtest(scipy.stats.ttest_ind, method='bonf')
result[0]
|
cs |
[output]
Test Multiple Comparison ttest_ind FWER=0.05
method=bonf alphacSidak=0.01, alphacBonf=0.008
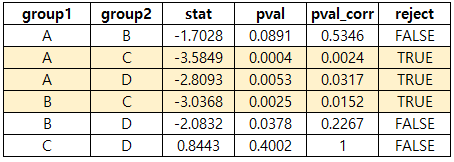
위의 결과를 해석해 보면,
A학급은 C, D 학급의 수학점수와 차이가 있고
B학급은 C학급과 수학점수의 유의차가 존재한다고 할수있다.
728x90
'IT > 파이썬' 카테고리의 다른 글
GridSearchCV 그리드서치 1탄 (0) | 2020.12.21 |
---|---|
의사결정나무(Decision Tree) 그래프 그리기 (0) | 2020.12.10 |
Imbalanced Dataset에서의 over sampling과 cross validation (0) | 2020.12.09 |
Chi-square test, Fisher's exact 검정 & post-hoc (0) | 2020.12.04 |
T-Test, levene test, 맨-휘트니 U 검정(Mann-Whitney U test) (0) | 2020.12.04 |